Wireless Systems Lab
Wireless technology has enormous potential to change the way people and things communicate. Future wireless networks will allow people on the move to communicate with anyone, anywhere, and at any time using a range of high-performance multimedia services. Wireless video will support applications such as enhanced social networking, distance learning and remote medicine. Wireless sensor networks can also enable a new class of intelligent home electronics, smart and energy-efficient buildings and highways, and in-body networks for analysis and treatment of medical conditions.
Fortunately for us researchers, there are many technical challenges that must be met in order to make this vision a reality. These challenges transcend all levels of the overall system design, including hardware, communication link design, wireless networking, distributed sensing, communication, and control, and cross-layer design. In addition, synergies between the hardware, link, and network designs must be exploited in order to meet the demanding performance requirements of these future systems.
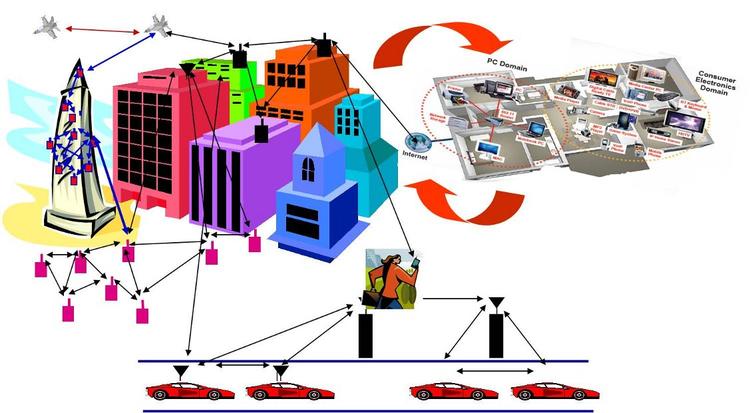
Current Research
Rethinking cellular system design
Next-generation cellular system will consist of an all-IP backbone network core with hierarchical macrocells, small cells, and indoor femtocells all sharing the same limited bandwidth. Optimal resource allocation, handoff, and WiFi offload is needed to achieve the Gbps data rates and near-ubiquitous coverage demanded by users. We are investigating self-organizing network techniques, timefrequency allocation, and handoffoffload mechanisms to achieve these ambitious performance goals.
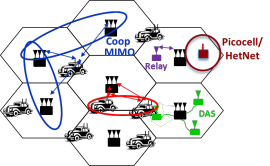
Physical-Layer Design for Future Generation Wireless Systems
We have developed the concept of “physical-layer cryptography” and proved that decoding massive MIMO signals can be cryptographically hard under certain conditions. This is accomplished by providing a Cook reduction from the same lattice problems that underly the Learning with Errors cryptosystem to decoding MIMO signals. We used this result to create a chosen-cyphertext secure (CCA) cryptosystem where the wireless channel plays the role of a private key. The resulting system is secure even in the presence of quantum computing.
We have developed and implemented highly efficient algorithms to perform blind decoding of MIMO signals (decoding without knowledge of the channel gain matrix). We expressed the problem of blind MIMO decoding as a non-convex optimization problem and showed that this problem may be solved efficiently through a combination of dynamic programing and by leveraging concepts from solving mixed-integer linear programs. This result will greatly reduce overhead from resource estimation, enable reliable MIMO decoding in time-varying channels, and potentially enable massive uncoordinated SIMO MAC or MU-MIMO deployments.
We are further interested in multicarrier modulation techniques for rapidly time-varying wireless channels. We have recently proposed an alternative to OFDM, named FDM-FDCP, which in many aspects is the time-frequency dual to OFDM. Our techniques are competitive with OFDM in terms of spectral efficiency and equalization complexity. Targeted applications of these techniques include mmWave systems, high-mobility environments (e.g. vehicle-to-vehicle communications, high-speed trains, etc.), and non-terrestrial environments (e.g. underwater, earth-to-space, etc.).
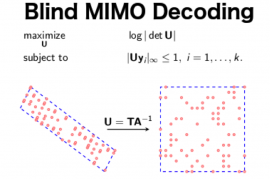
Capacity of wireless channels and networks
One of the fundamental issues in the design of a wireless communication system is how fast data can be transmitted reliably. The theoretical upper bound for speed of data transmission over a channel is called the channel capacity. The capacity of time-varying channels depends on what is known about the channel at the transmitter and/or receiver. It also depends on whether rate can be adaptive or constant, as well as whether or not service outage can be tolerated. We have investigated capacity and capacity regions of time-varying multiuser channels, cellular systems, and ad-hoc networks with one or more antennas at the transmitter(s) and receiver(s).
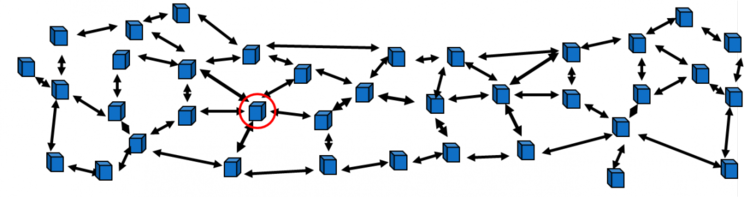
Software-defined wireless networks
Software-defined wireless networks provide a unified control plane for wireless hardware to dynamically optimize heterogeneous hardware across multiple spectral bands. The control plane allows for dynamic configuration of radio parameters such as channel, power control, antenna parameters to minimize interference and provide the required performance to heterogenous applications. The control plane can also provide seamless handoff between different wireless networks as well as utilize multiple networks simultaneously to provide robustness as required for demanding applications such as video and real-time control.
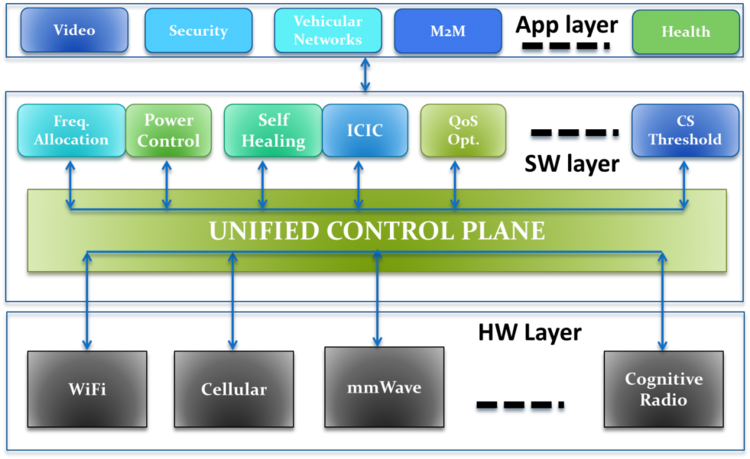
Distributed sensing, communications, and control
This area of research focuses on the interplay between control and communication, optimal sparse sensing and state estimation for high dimensional control systems, as well as sensor placement, outage detection and cyber physical security. We looked at the problem of estimating high-dimensional autoregressive time series from measurements arising from a large number of distributed sensors. Communication or computational constraints may lead to collection of sampled noisy measurements; we investigated estimation performance with this corruption and also observed how placing structural constraints on the time series improves performance. Future directions would include performing this estimation in a distributed setting or controlling and simultaneously learning high-dimensional dynamical systems.
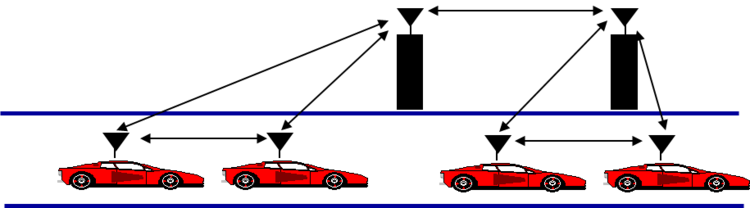
Bio-inspired forms of communication using chemical and molecular signals
In this paradigm of molecular communication, the transmitter releases chemicals or molecules, and encodes information on some property of these signals such as their timing or concentration. The signal then propagates through the medium between the transmitter and the receiver via means such as diffusion, until it arrives at the receiver where the signal is detected and the information decoded. This new multidisciplinary field can be used for in-body communication, secrecy, networking microscale and nanoscale devices, infrastructure monitoring in smart cities and industrial complexes, as well as for underwater communications. Since these systems are fundamentally different from telecommunication systems, most techniques that have been developed over the past few decades to advance radio technology cannot be applied to them directly. This research is on how to use chemical and molecular signals to form networks of different scales (from kilometers to nanometers) by both adapting methods from modern telecommunications, and developing new techniques.
Communications and signal processing in bioengineering and neuroscience
We develop signal processing algorithms for neuroscience, with a focus on epilepsy, one of the most common neurological disorders. While in most cases treating epilepsy with antiepileptic drugs is successful, this is not effective for about a third of the patients. The main treatment for such patients is a surgical procedure for removal of the seizure onset zone (SOZ), the area in the brain from which the seizures originate. The main tool for accurately identifying the SOZ is electrocorticography (ECoG) recordings taken from grids or strips of electrodes placed on the cortex. In this research we design algorithms for SOZ localization, detection and prediction of epileptic seizures, using ECoG recordings. We use tools from communication theory, signal processing and statistical signal processing, control theory, information theory, machine learning, graphs theory, and time-series analysis.
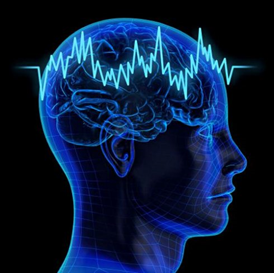
We have also applied signal processing methods to separate gene expression microarray mixed cell tissue samples. For example, tumor biopsy tissues that are profiled using microarrays contain a mixture of tumor cells, infiltrating immune cells and additional microenvironmental cells such as fibroblasts. Previous studies have shown that microarray measurements are well modeled as a linear mixture of multiple signatures from different cell types. This problem is almost identical to signal processing of hyper-spectral imaging. Thus, by incorporating state of the art signal processing tools we successfully identify the cell types in the mixture, extract their individual signatures and relative proportions per sample (Zuckerman et al. PLoS Comput Biol. 2013;9(8):e1003189). Application of this method to the abundance of gene expression microarray samples that exist in publicly available repositories can be a powerful tool for the discovery of phenomena otherwise not detected in the mixed tissue samples of individual experiments.
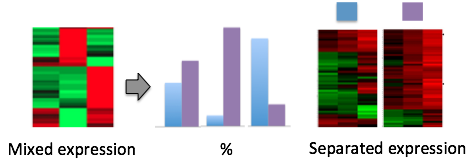